Predictive Maintenance of Machines
- Advance Technologies
- IT Services
- Machine Learning
- Manufacturing
- Predictive Maintenance
- Resource Planning
- Transportation
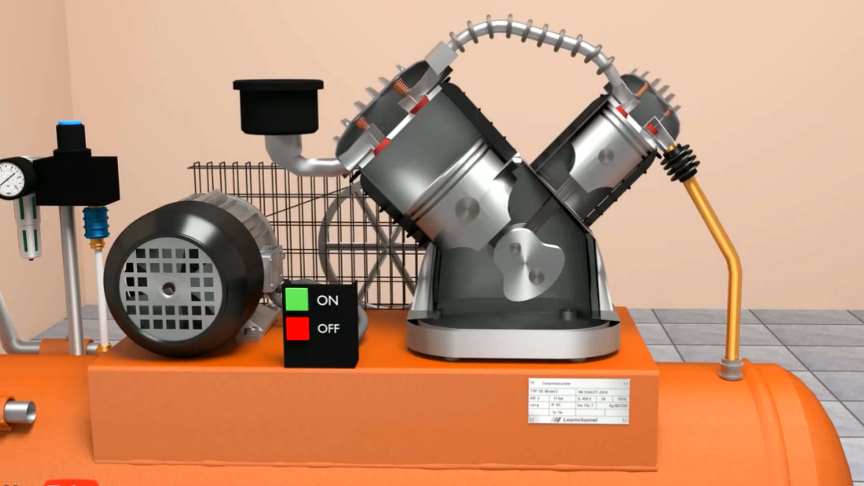
If you operate in industries such as manufacturing or transportation, where the continuous operation of critical machinery is vital for day-to-day productivity, any machine failure can result in disruptions, leading to not only repair costs but also revenue losses. An example shared by a principal data scientist responsible for locomotives at a railway illustrates the impact of predictive capabilities. They reported saving $5,000,000 in revenues alone, along with an additional $2,000,000 in costs by leveraging machine learning (ML) models that predict failures well in advance.
So, how is it all accomplished? Let’s take a closer look at the process:
1. Begin by gathering relevant data from the machines and their sensors. Discuss the data with the stakeholders to gain a comprehensive understanding of the machines and processes involved. Employed common sense coupled with the appropriate data science approaches during this phase. Data and its quality play a critical role for the success of ML predictions, so allocate sufficient time for data collection and perform necessary data preparation processes before initiating the ML process. This entails a robust ETL (Extract, Transform, and Load) process to address data quality issues.
2. Proceed with exploratory data analysis, feature engineering, selection, model building and tuning. Carefully select the model that performs well on unseen data and deploy it. This completes the data science and ML process.
3. Constantly monitor and evaluate models to account for any drifts in both the models and data, ensuring the quality and reliability of predictions over time. Consider implementation factors such as system scalability, low latency, and costs.
By following this predictive maintenance process using ML, businesses can minimize revenues losses and reduce expenses. It’s crucial to continuously assess and improve the models to maintain their effectiveness. The scalability, low latency, and cost efficiency of the implemented system should also be considered.